Recommendation Tips About How To Avoid Multicollinearity
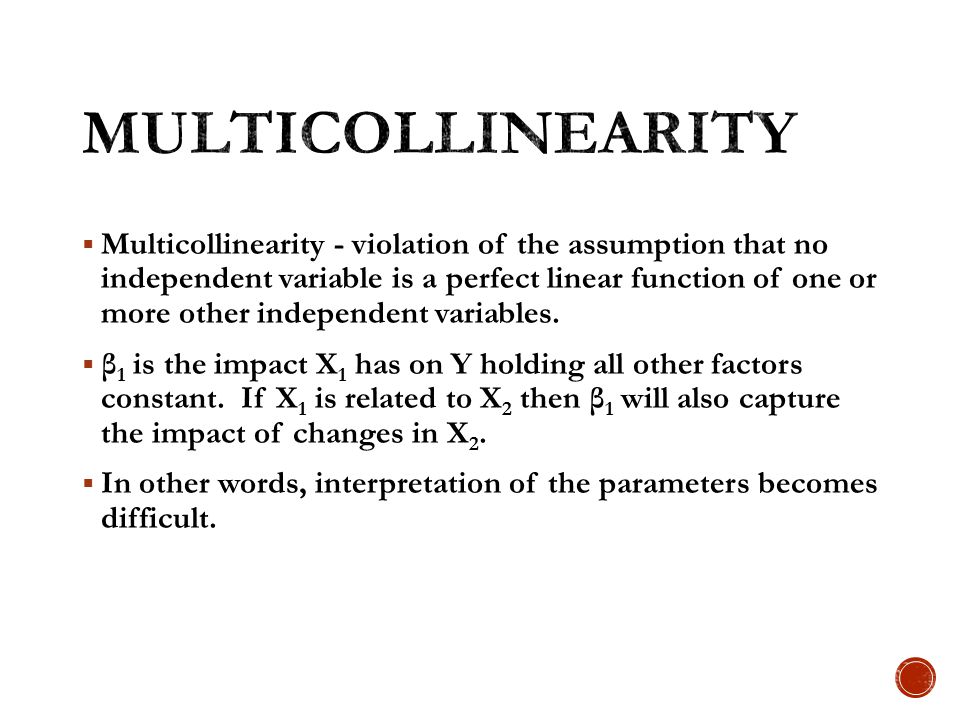
In this tutorial, we will walk through a simple example on how you can deal with the multi.
How to avoid multicollinearity. There are multiple ways to overcome the problem of multicollinearity. To determine if multicollinearity is a problem, we can produce vif values for each of the predictor variables. A special solution in polynomial models is.
Pd.get_dummies silently introduces multicollinearity in your data. To do so, click on the analyze tab, then regression , then linear : You may use ridge regression or principal component regression or partial least squares regression.
Using vif (variation inflation factor) 1. Multicollinearity is a problem in polynomial regression (with terms of second and higher order): If there is only moderate multicollinearity, you likely.
To reduce the amount of multicollinearity found in a model, one can remove the specific variables that are identified as the most collinear. In general, there are two different methods to remove multicollinearity — 1. Hence, greater vif denotes greater correlation.
[this was directly from wikipedia]. This is in agreement with the fact that a higher. X and x^2 tend to be highly correlated.
Fortunately, it’s possible to detect multicollinearity using a metric known as the variance inflation factor (vif), which measures the correlation and strength of correlation.